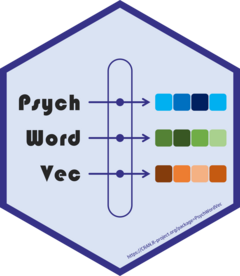
Train static word embeddings using the Word2Vec, GloVe, or FastText algorithm.
Source:R/02-static.R
train_wordvec.Rd
Usage
train_wordvec(
text,
method = c("word2vec", "glove", "fasttext"),
dims = 300,
window = 5,
min.freq = 5,
threads = 8,
model = c("skip-gram", "cbow"),
loss = c("ns", "hs"),
negative = 5,
subsample = 1e-04,
learning = 0.05,
ngrams = c(3, 6),
x.max = 10,
convergence = -1,
stopwords = character(0),
encoding = "UTF-8",
tolower = FALSE,
normalize = FALSE,
iteration,
tokenizer,
remove,
file.save,
compress = "bzip2",
verbose = TRUE
)
Arguments
- text
A character vector of text, or a file path on disk containing text.
- method
Training algorithm:
- dims
Number of dimensions of word vectors to be trained. Common choices include 50, 100, 200, 300, and 500. Defaults to
300
.- window
Window size (number of nearby words behind/ahead the current word). It defines how many surrounding words to be included in training: [window] words behind and [window] words ahead ([window]*2 in total). Defaults to
5
.- min.freq
Minimum frequency of words to be included in training. Words that appear less than this value of times will be excluded from vocabulary. Defaults to
5
(take words that appear at least five times).- threads
Number of CPU threads used for training. A modest value produces the fastest training. Too many threads are not always helpful. Defaults to
8
.- model
<Only for Word2Vec / FastText>
Learning model architecture:
"skip-gram"
(default): Skip-Gram, which predicts surrounding words given the current word"cbow"
: Continuous Bag-of-Words, which predicts the current word based on the context
- loss
<Only for Word2Vec / FastText>
Loss function (computationally efficient approximation):
"ns"
(default): Negative Sampling"hs"
: Hierarchical Softmax
- negative
<Only for Negative Sampling in Word2Vec / FastText>
Number of negative examples. Values in the range 5~20 are useful for small training datasets, while for large datasets the value can be as small as 2~5. Defaults to
5
.- subsample
<Only for Word2Vec / FastText>
Subsampling of frequent words (threshold for occurrence of words). Those that appear with higher frequency in the training data will be randomly down-sampled. Defaults to
0.0001
(1e-04
).- learning
<Only for Word2Vec / FastText>
Initial (starting) learning rate, also known as alpha. Defaults to
0.05
.- ngrams
<Only for FastText>
Minimal and maximal ngram length. Defaults to
c(3, 6)
.- x.max
<Only for GloVe>
Maximum number of co-occurrences to use in the weighting function. Defaults to
10
.- convergence
<Only for GloVe>
Convergence tolerance for SGD iterations. Defaults to
-1
.- stopwords
<Only for Word2Vec / GloVe>
A character vector of stopwords to be excluded from training.
- encoding
Text encoding. Defaults to
"UTF-8"
.- tolower
Convert all upper-case characters to lower-case? Defaults to
FALSE
.- normalize
Normalize all word vectors to unit length? Defaults to
FALSE
. Seenormalize
.- iteration
Number of training iterations. More iterations makes a more precise model, but computational cost is linearly proportional to iterations. Defaults to
5
for Word2Vec and FastText while10
for GloVe.- tokenizer
Function used to tokenize the text. Defaults to
text2vec::word_tokenizer
.- remove
Strings (in regular expression) to be removed from the text. Defaults to
"_|'|<br/>|<br />|e\\.g\\.|i\\.e\\."
. You may turn off this by specifyingremove=NULL
.- file.save
File name of to-be-saved R data (must be .RData).
- compress
Compression method for the saved file. Defaults to
"bzip2"
.Options include:
1
or"gzip"
: modest file size (fastest)2
or"bzip2"
: small file size (fast)3
or"xz"
: minimized file size (slow)
- verbose
Print information to the console? Defaults to
TRUE
.
Download
Download pre-trained word vectors data (.RData
):
https://psychbruce.github.io/WordVector_RData.pdf
Examples
review = text2vec::movie_review # a data.frame'
text = review$review
## Note: All the examples train 50 dims for faster code check.
## Word2Vec (SGNS)
dt1 = train_wordvec(
text,
method="word2vec",
model="skip-gram",
dims=50, window=5,
normalize=TRUE)
#> ✔ Tokenized: 70105 sentences (time cost = 3 secs)
#> ✔ Text corpus: 5242249 characters, 1185427 tokens (roughly words)
#>
#> ── Training model information ──────────────────────────────────────────────────
#> - Method: Word2Vec (Skip-Gram with Negative Sampling)
#> - Dimensions: 50
#> - Window size: 5 (5 words behind and 5 words ahead the current word)
#> - Subsampling: 1e-04
#> - Min. freq.: 5 occurrences in text
#> - Iterations: 5 training iterations
#> - CPU threads: 8
#>
#> ── Training...
#> ✔ Word vectors trained: 14205 unique tokens (time cost = 11 secs)
dt1
#> # wordvec (data.table): [14205 × 3] (normalized)
#> word vec freq
#> 1: the [ 0.1822, ...<50 dims>] 58797
#> 2: and [ 0.1831, ...<50 dims>] 32193
#> 3: a [-0.0734, ...<50 dims>] 31783
#> 4: of [ 0.0264, ...<50 dims>] 29142
#> 5: to [-0.0123, ...<50 dims>] 27218
#> ------
#> 14201: parrot [ 0.2487, ...<50 dims>] 5
#> 14202: Lori [ 0.1793, ...<50 dims>] 5
#> 14203: shambles [ 0.1917, ...<50 dims>] 5
#> 14204: comprehension [ 0.1882, ...<50 dims>] 5
#> 14205: drunks [ 0.2310, ...<50 dims>] 5
most_similar(dt1, "Ive") # evaluate performance
#> [Word Vector] =~ Ive
#> (normalized to unit length)
#> word cos_sim row_id
#> 1: ive 0.8520610 110
#> 2: seen 0.7974154 1542
#> 3: lately 0.7785641 2573
#> 4: Youve 0.7734673 2644
#> 5: Guinea 0.7710928 3125
#> 6: weve 0.7620411 3487
#> 7: recall 0.7611667 5137
#> 8: scarier 0.7581943 5989
#> 9: funniest 0.7571287 9121
#> 10: quote 0.7567139 9820
most_similar(dt1, ~ man - he + she, topn=5) # evaluate performance
#> [Word Vector] =~ man - he + she
#> (normalized to unit length)
#> word cos_sim row_id
#> 1: woman 0.8116181 260
#> 2: girl 0.7620174 299
#> 3: widow 0.7290366 478
#> 4: boy 0.7268918 523
#> 5: child 0.7171464 5485
most_similar(dt1, ~ boy - he + she, topn=5) # evaluate performance
#> [Word Vector] =~ boy - he + she
#> (normalized to unit length)
#> word cos_sim row_id
#> 1: girl 0.8339958 150
#> 2: woman 0.7191492 260
#> 3: kid 0.6743736 299
#> 4: aged 0.6560675 674
#> 5: old 0.6471804 1839
## GloVe
dt2 = train_wordvec(
text,
method="glove",
dims=50, window=5,
normalize=TRUE)
#> ✔ Tokenized: 70105 sentences (time cost = 2 secs)
#> ✔ Text corpus: 5242249 characters, 1185427 tokens (roughly words)
#>
#> ── Training model information ──────────────────────────────────────────────────
#> - Method: GloVe
#> - Dimensions: 50
#> - Window size: 5 (5 words behind and 5 words ahead the current word)
#> - Subsampling: N/A
#> - Min. freq.: 5 occurrences in text
#> - Iterations: 10 training iterations
#> - CPU threads: 8
#>
#> ── Training...
#> ✔ Word vectors trained: 14207 unique tokens (time cost = 13 secs)
dt2
#> # wordvec (data.table): [14207 × 3] (normalized)
#> word vec freq
#> 1: the [ 0.0626, ...<50 dims>] 58797
#> 2: and [ 0.0975, ...<50 dims>] 32193
#> 3: a [ 0.0044, ...<50 dims>] 31783
#> 4: of [ 0.0567, ...<50 dims>] 29142
#> 5: to [ 0.0567, ...<50 dims>] 27218
#> ------
#> 14203: yea [ 0.1668, ...<50 dims>] 5
#> 14204: yearly [-0.0390, ...<50 dims>] 5
#> 14205: yearning [-0.0154, ...<50 dims>] 5
#> 14206: yelled [ 0.2584, ...<50 dims>] 5
#> 14207: yer [-0.0821, ...<50 dims>] 5
most_similar(dt2, "Ive") # evaluate performance
#> [Word Vector] =~ Ive
#> (normalized to unit length)
#> word cos_sim row_id
#> 1: seen 0.9426213 74
#> 2: ever 0.8902116 110
#> 3: heard 0.7670113 124
#> 4: worst 0.7562863 261
#> 5: since 0.7308189 262
#> 6: youve 0.7038168 305
#> 7: watched 0.6943821 468
#> 8: been 0.6859998 515
#> 9: already 0.6812158 767
#> 10: havent 0.6796917 950
most_similar(dt2, ~ man - he + she, topn=5) # evaluate performance
#> [Word Vector] =~ man - he + she
#> (normalized to unit length)
#> word cos_sim row_id
#> 1: woman 0.8636718 34
#> 2: young 0.7500165 198
#> 3: hit 0.7368818 260
#> 4: who 0.7318680 299
#> 5: girl 0.7256584 594
most_similar(dt2, ~ boy - he + she, topn=5) # evaluate performance
#> [Word Vector] =~ boy - he + she
#> (normalized to unit length)
#> word cos_sim row_id
#> 1: girl 0.8009999 198
#> 2: young 0.7494713 260
#> 3: named 0.7147159 299
#> 4: woman 0.7000530 675
#> 5: kid 0.6682569 867
## FastText
dt3 = train_wordvec(
text,
method="fasttext",
model="skip-gram",
dims=50, window=5,
normalize=TRUE)
#> ✔ Tokenized: 70105 sentences (time cost = 2 secs)
#> ✔ Text corpus: 5242249 characters, 1185427 tokens (roughly words)
#>
#> ── Training model information ──────────────────────────────────────────────────
#> - Method: FastText (Skip-Gram with Negative Sampling)
#> - Dimensions: 50
#> - Window size: 5 (5 words behind and 5 words ahead the current word)
#> - Subsampling: 1e-04
#> - Min. freq.: 5 occurrences in text
#> - Iterations: 5 training iterations
#> - CPU threads: 8
#>
#> ── Training...
#> ✔ Word vectors trained: 14207 unique tokens (time cost = 22 secs)
dt3
#> # wordvec (data.table): [14207 × 3] (normalized)
#> word vec freq
#> 1: the [-0.0067, ...<50 dims>] 58797
#> 2: and [ 0.0646, ...<50 dims>] 32193
#> 3: a [ 0.0955, ...<50 dims>] 31783
#> 4: of [ 0.0448, ...<50 dims>] 29142
#> 5: to [-0.1173, ...<50 dims>] 27218
#> ------
#> 14203: spray [ 0.1319, ...<50 dims>] 5
#> 14204: disabilities [ 0.0282, ...<50 dims>] 5
#> 14205: crook [ 0.0777, ...<50 dims>] 5
#> 14206: Syndrome [ 0.0494, ...<50 dims>] 5
#> 14207: snipers [ 0.0040, ...<50 dims>] 5
most_similar(dt3, "Ive") # evaluate performance
#> [Word Vector] =~ Ive
#> (normalized to unit length)
#> word cos_sim row_id
#> 1: Youve 0.8312521 110
#> 2: Weve 0.8151651 765
#> 3: seen 0.8071763 945
#> 4: youve 0.7793227 3105
#> 5: WORST 0.7651692 3250
#> 6: ve 0.7601260 5898
#> 7: funnier 0.7528114 6913
#> 8: beforehand 0.7424984 7108
#> 9: Columbo 0.7380933 9171
#> 10: havent 0.7351666 12894
most_similar(dt3, ~ man - he + she, topn=5) # evaluate performance
#> [Word Vector] =~ man - he + she
#> (normalized to unit length)
#> word cos_sim row_id
#> 1: woman 0.8809123 261
#> 2: girl 0.7759282 299
#> 3: salesman 0.7683804 5594
#> 4: madman 0.7671942 6553
#> 5: henchman 0.7638086 12263
most_similar(dt3, ~ boy - he + she, topn=5) # evaluate performance
#> [Word Vector] =~ boy - he + she
#> (normalized to unit length)
#> word cos_sim row_id
#> 1: girl 0.7678865 261
#> 2: woman 0.7330712 299
#> 3: kid 0.6992820 676
#> 4: boys 0.6859984 1045
#> 5: teenager 0.6849031 2364